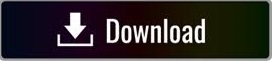
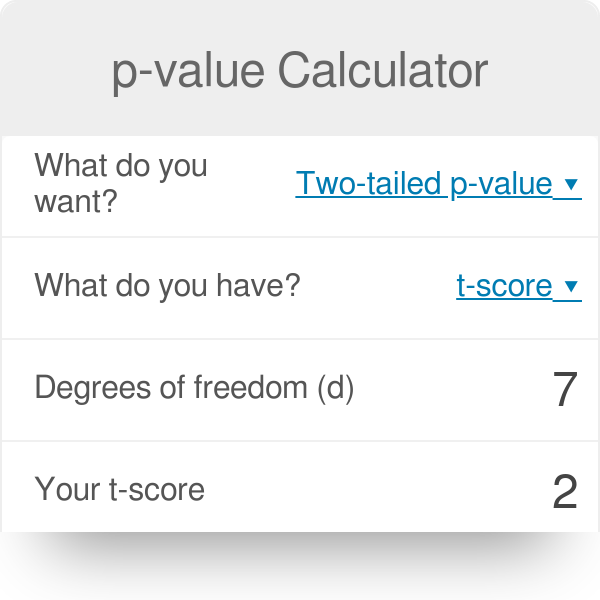
The output tells us that the average Brinell hardness of the n = 25 pieces of ductile iron was 172.52 with a standard deviation of 10.31. Otherwise, we fail to reject the null.Alternative hypothesis H₁: $\mu$ > 170 T-Value If we find a result that clears the bar we’ve set for ourselves, then we reject the null hypothesis and we say that the finding is significant at the ?p?-value that we find. And therefore there’s only a ?1? in ?100? chance that we’ll reject the null hypothesis when we really shouldn’t have, thinking that we provided support for the alternative hypothesis when we shouldn’t have. At that significance level, there’s only a ?1? in ?100? chance that the result we got was just by chance. And therefore there’s a ?1? in ?10? chance that we’ll reject the null hypothesis when we really shouldn’t have, thinking that we provided support for the alternative hypothesis when we shouldn’t have.īut a stricter alpha level of ?0.01? (or a ?p?-value of ?0.01?, or a confidence level of ?99\%?) is a higher bar to clear. At that significance level, there’s a ?1? in ?10? chance that the result we got was just by chance. In other words, an alpha level of ?0.10? (or a ?p?-value of ?0.10?, or a confidence level of ?90\%?) is a lower bar to clear. The smaller the ?p?-value, or the smaller the alpha value, or the lower the Type I error rate, and the smaller the region of rejection, the higher the confidence level, and the less likely it is that you got your result by chance. There’s a ?1? in ?100? chance of getting a result as, or more, extreme as this one The area of the rejection region is ?0.01? The finding is significant at the ?0.01? level
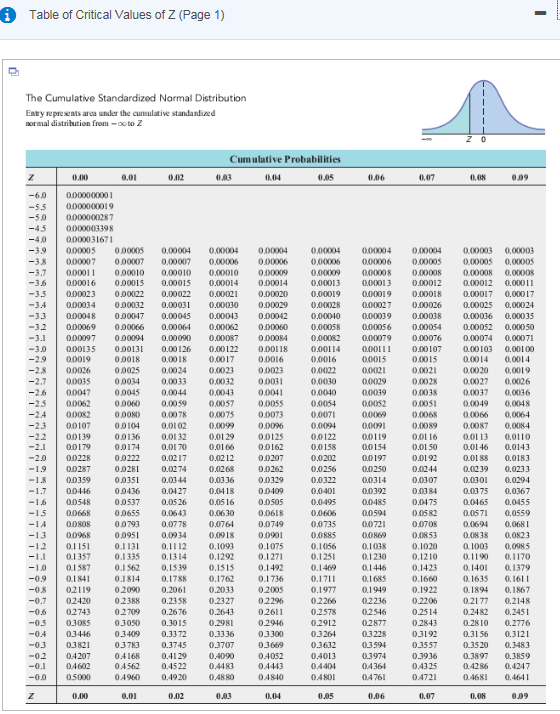
Hopefully by now it’s not too surprising by now that all of these are equivalent statements: The less likely it is that we obtained a result by chance, the more significant our results. The significance (or statistical significance) of a test is the probability of obtaining your result by chance. If, however, we’d picked a more rigorous ?\alpha=0.05? or ?\alpha=0.01?, we would have failed to reject the null hypothesis every time. So we would have rejected the null hypothesis for both one-tailed tests, but we would have failed to reject the null in the two-tailed test. With these in mind, let’s say for instance you set the confidence level of your hypothesis test at ?90\%?, which is the same as setting the ?\alpha? level at ?\alpha=0.10?. ?p=0.0721? for the upper-tail one-tailed test ?p=0.0721? for the lower-tail one-tailed test If ?p>\alpha?, do not reject the null hypothesis If ?p\leq \alpha?, reject the null hypothesis
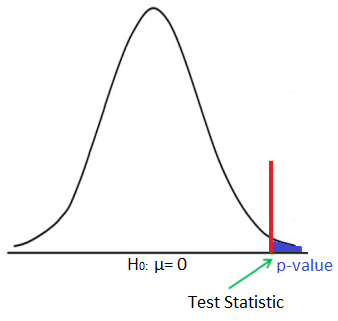
Whether or not you should reject ?H_0? can be determined by the relationship between the ?\alpha? level and the ?p?-value. The reason we’ve gone through all this work to understand the ?p?-value is because using a ?p?-value is a really quick way to decide whether or not to reject the null hypothesis.
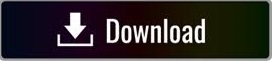